International Journal of Progressive Research in Engineering Management and Science
(Peer-Reviewed, Open Access, Fully Referred International Journal)
www.ijprems.com
editor@ijprems.com or Whatsapp at (+91-9098855509)
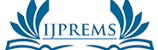
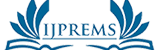
A Review of Neural Network Compression and Pruning using Shapley Pruning (KEY IJP************477)
Abstract
Neural network compression and pruning are two of the most vital methodologies that would make these deep architectures efficient so they can be deployed on these low-resource devices without significant deterioration in the chances of accuracy. Deep learning models are increasingly dominating NLP, computer vision, and healthcare applications and thus have witnessed an exponential increase in the related computational demands on memory for this model. This reduces the model sizes because either the precision of weight is downgraded or complexity in architecture is streamlined. In contrast, pruning results in the removal of excess weights, neurons, or even layer targeting the less impactful components a neural network. These optimizations resulted in better inference speed as well as lesser energy consumption which are crucial for this deployment in mobile platforms, Internet of things devices, and edge-computing systems. However, one of the challenges of this model simplification is the balance between efficiency and performance. Aggressive pruning and compression yield accuracy loss. Recent work on hardware-aware pruning, dynamic sparsity, and automated architecture design via NAS attempts to solve these challenges to produce more adaptive and high-performance models. Advancements in neural network compression and pruning are eyed to increase the scalability and sustainability of artificial intelligence technologies. These developments are expected to increase penetration across industries while helping the sector meet a growing need for energy efficiency in AI solutions.
DOI Requested