International Journal of Progressive Research in Engineering Management and Science
(Peer-Reviewed, Open Access, Fully Referred International Journal)
www.ijprems.com
editor@ijprems.com or Whatsapp at (+91-9098855509)
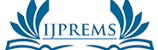
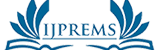
Boston Housing Prediction Using Machine Learning (KEY IJP************237)
Abstract
Boston Housing Prediction Regression is a well-known example in machine learning where the goal is to predict house prices in Boston based on various features. This problem is tackled using the well-known Boston Housing Dataset, which includes variables such as crime rate, property tax, number of rooms, accessibility to highways, crime rate in the neighbourhood, distance to employment centers, availability of schools, and other related aspects. The objective is to build a predictive model that can estimate house prices by finding a relationship between these features and the price.This is useful for potential buyers, investors, and real estate analysts to understand how different factors influence housing prices in the Boston area. By using this dataset, one can learn how attributes like crime rates, proximity to healthcare or schools, and property age impact the market value. The Boston Housing dataset is often used as a foundation to practice and learn about predictive models in data science and serves as an important tool for making informed decisions in the real estate market. This paper applies data preprocessing, feature engineering, and different regression techniques to build a predictive model. Various algorithms, including Linear Regression, Decision Trees, and Random Forest, are evaluated for their performance. Additionally, more advanced techniques like XG Boost and can be explored to improve predictive accuracy. The model is assessed using metrics like Mean Squared Error (MSE) and Cross-Validation Score to ensure high accuracy and reliability.Feature selection plays a crucial role in refining the models performance by eliminating irrelevant or redundant variables. Data normalization and transformation techniques are also applied to enhance the efficiency of regression models.. The insights from this study can assist home buyers, real estate agencies, and policymakers in making data-driven decisions.
DOI Requested