International Journal of Progressive Research in Engineering Management and Science
(Peer-Reviewed, Open Access, Fully Referred International Journal)
www.ijprems.com
editor@ijprems.com or Whatsapp at (+91-9098855509)
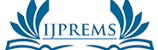
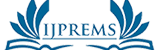
AGRICULTURAL MARKETING AND SALES (KEY IJP************218)
Abstract
Agricultural marketing and sales forecasting are critical for optimizing agricultural production and enhancing market efficiency. This study presents a comprehensive approach to predicting crop yields and market prices using machine learning models. By integrating environmental factors such as weather conditions, soil quality, and historical data on crop yields, the model forecasts cultivation outcomes. Additionally, economic variables like demand, supply, and historical pricing trends are considered to predict future market prices for crops. This dual prediction framework aims to assist farmers and agricultural stakeholders in making informed decisions about planting, harvesting, and selling crops, ultimately improving profitability and reducing risks associated with market volatility. The model's performance is evaluated using real-world agricultural datasets, and the results indicate a significant improvement in prediction accuracy compared to traditional methods.Keywords: Crop prediction, yield forecasting, machine learning, sales price prediction, agricultural analytics, market forecasting.1. INTRODUCTION In agriculture, Agricultural marketing and sales is essential for maximizing productivity and profitability. Agricultural marketing and sales estimates expected yields based on factors like weather, soil quality, and historical performance, helping farmers make informed planting decisions. On the other hand, sales price prediction involves forecasting market prices using economic indicators such as supply, demand, and past trends. Accurate predictions of both crop yields and prices enable farmers to optimize production, manage risks, and make strategic decisions about when to sell their produce. By leveraging advanced technologies like machine learning, these predictions can significantly improve farm efficiency and financial outcomes. Crop cultivation prediction involves estimating the expected yield of crops based on factors such as weather patterns, soil conditions, irrigation, and historical crop performance. By leveraging advanced technologies, such as machine learning and big data analytics, farmers and agribusinesses can optimize their planting strategies, allocate resources effectively, and maximize crop yields. Accurate crop predictions not only help in managing the production cycle but also reduce the risk of crop failure due to unforeseen environmental conditions.Equally crucial is the ability to forecast sales prices for crops. Price volatility in agricultural markets can significantly impact farmersprofitability and the stability of the food supply chain. Sales price prediction models incorporate various economic factors, such as supply and demand, global trade dynamics, and historical pricing trends, to provide farmers with actionable insights on when to sell their produce for maximum profit. Predicting market prices enables better financial planning and helps farmers avoid losses due to unfavorable market conditions. This paper focuses on combining crop yield prediction with sales price forecasting to develop a comprehensive decision-making tool for farmers and agricultural stakeholders. By utilizing machine learning algorithms and integrating both environmental and economic variables, the proposed framework aims to enhance precision agriculture, improve farm profitability, and contribute to sustainable farming practices.