International Journal of Progressive Research in Engineering Management and Science
(Peer-Reviewed, Open Access, Fully Referred International Journal)
www.ijprems.com
editor@ijprems.com or Whatsapp at (+91-9098855509)
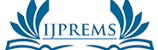
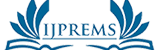
Curating Ideal Datasets for Parkinson's disease Detection with Machine Learning Techniques (KEY IJP************011)
Abstract
A neurodegenerative disorder called Parkinson's disease primarily affects the dopamine-producing ("dopaminergic") neurons in a specific area of the brain called the substantia. The symptoms can be either movement-related (or "motor"), such as postural instability, trouble walking, speech problems, swallowing difficulties, etc., or they can be non-motor (or unconnected to movement). The onset of Parkinson's disease typically occurs at age 60 and naturally rises with age. While treatments and medications can lessen symptoms, there is no known cure. PD has doubled during the last 25 years, according to the WHO. Feed forward neural networks (FNN), a type of machine learning (ML) approach, have demonstrated significant promise in improving diagnostic accuracy. FNN performance is highly dependent on the caliber and applicability of the datasets employed. Effective feature extraction methods specific to PD datasets are evaluated in this study, with a view on significance of choosing the relevant features, such as tremor frequency, gait abnormalities, speech abnormalities, and patient demographics, as these factors have a direct influence on FNN learning ability. The study looks at data pre-processing methods such dimensionality reduction, feature scaling, and normalization to improve the model's effectiveness with 95% accuracy. We show through comparative analysis how well selected datasets can greatly increase the accuracy of PD identification. Our research aids researchers and medical professionals studying neurodegenerative illnesses create more trustworthy machine learning tools.
DOI Requested