International Journal of Progressive Research in Engineering Management and Science
(Peer-Reviewed, Open Access, Fully Referred International Journal)
www.ijprems.com
editor@ijprems.com or Whatsapp at (+91-9098855509)
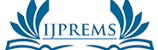
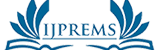
BRAIN TUMOR ANALYSATION USING DEEP LEARNING METHODS (KEY IJP************456)
Abstract
Brain tumor detection in its early stages is critical for effective treatment and improved patient outcomes. This study explores the application of machine learning (ML), deep learning (DL), and hybrid classifiers to accurately detect and classify brain tumors using Magnetic Resonance (MR) images. A comparative analysis of different classifiers was conducted to identify the most effective approach.The MR images were preprocessed using skull stripping to eliminate non-relevant details and segmented using K-means clustering to isolate the tumor region. Features were extracted using the Gray Level Co-occurrence Matrix (GLCM) method, which provided valuable numerical data for classification. Eight ML classifiers, including Decision Tree, Support Vector Machine (SVM), k-Nearest Neighbor (kNN), and Naive Bayes, were evaluated based on metrics such as accuracy, sensitivity, and specificity.DL classifiers, such as Convolutional Neural Networks (CNN), were employed to automate feature extraction and enhance the diagnostic process. However,standalone DL models demonstrated lower accuracy compared to ML classifiers due to challenges in handling feature complexities. To combine the strengths of both approaches, a hybrid model integrating a Deep Neural Network (DNN) with ML classifiers was developed.
DOI Requested