International Journal of Progressive Research in Engineering Management and Science
(Peer-Reviewed, Open Access, Fully Referred International Journal)
www.ijprems.com
editor@ijprems.com or Whatsapp at (+91-9098855509)
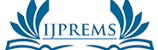
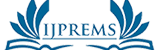
An In-Depth Analysis of Machine Learning Models for Accurate Crop Yield Prediction (KEY IJP************263)
Abstract
Machine learning is an emerging field in crop yield prediction, playing a pivotal role in modern agriculture. Accurately forecasting crop yield is essential for farmers to estimate their harvest. Traditionally, yield predictions were based on a farmers experience with specific crops and fields, but achieving precise predictions from available data has been a significant challenge. Machine learning offers a promising solution to this problem. Several machine learning techniques have been explored and evaluated to predict crop production for upcoming seasons. This paper proposes a system that predicts crop yield using historical data, leveraging machine learning algorithms like Support Vector Machine (SVM) and Random Forest. Additionally, it provides crop-specific fertilizer recommendations. The paper focuses on developing a predictive model that can forecast crop yield and serve as a tool for future agricultural planning. The project aims to build a machine learning-powered web application to assist farmers in making informed decisions about crop cultivation. By analyzing factors such as land type, budget, and soil characteristics, the system offers tailored recommendations on suitable crops, fertilizers, and pesticides. It also alerts farmers to potential crop diseases due to inadequate care and provides insights into soil pH, nutrient levels, and water needs. Ultimately, this project seeks to enhance agricultural productivity by accurately predicting yields and profits, helping farmers optimize their output while mitigating risks.