International Journal of Progressive Research in Engineering Management and Science
(Peer-Reviewed, Open Access, Fully Referred International Journal)
www.ijprems.com
editor@ijprems.com or Whatsapp at (+91-9098855509)
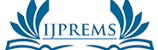
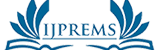
Optimizing Object Detection in Autonomous Driving: Leveraging YOLO Algorithms (KEY IJP************520)
Abstract
In the future, autonomous driving systems will require accurate object detection to guarantee safety and efficiency. In this paper, we will assess YOLOv7 and YOLOv9, two state-of-the-art deep learning algorithms, for real-time object detection in such vehicles. We will test their performance on a high precision dataset measuring simultaneously their accuracy, speed and robustness in various scenarios. First, an overview of the architecture of the YOLO algorithm is described regarding its impact on real-time detection for autonomous driving. The more objects that the network has to detect the longer it takes due to bounding box regression overheads per image. Also, recognition predicts categories not locations or attributes so there is naturally a blind spot where objects are small or unusual. Our results indicate that YOLOv9 outperforms YOLOv7 with respect to detecting larger as well as smaller objects while YOLOv7 is better suited for detecting small objects. Therefore we propose to cascade both in order measure every aspect of interest within a frame leading to an advancement in autonomous driving.Keywords: Autonomous driving, object Detection, YOLOv7, YOLOv9, deep learning, computer vision