International Journal of Progressive Research in Engineering Management and Science
(Peer-Reviewed, Open Access, Fully Referred International Journal)
www.ijprems.com
editor@ijprems.com or Whatsapp at (+91-9098855509)
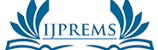
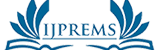
AI-DRIVEN MEDICAL IMAGING FOR EARLY DETECTION OF KIDNEY STONES (KEY IJP************985)
Abstract
Kidney stone disease, a common and painful condition, poses significant challenges for accurate and timely diagnosis. Traditional diagnostic methods, including CT scans, ultrasound, and X-rays, often suffer from limitations such as high costs, radiation exposure, and subjective interpretation, which can result in missed or delayed diagnoses. Recent advancements in artificial intelligence, particularly Convolutional Neural Networks (CNNs), offer a promising solution to enhance kidney stone detection and classification from medical images. This research aims to develop an automated kidney stone detection system using CNNs, ReLU activation functions, and the SGD optimizer. By processing CT images and addressing issues such as noise and image quality, the system intends to improve diagnostic accuracy, reduce the workload of healthcare professionals, and provide faster, more consistent results. The study explores the potential of CNNs for image analysis, feature extraction, and classification, and evaluates the system's performance in terms of sensitivity, specificity, and computational efficiency. The findings have significant implications for improving clinical decision-making, reducing healthcare costs, and enhancing patient outcomes. This research contributes to the growing field of AI in medical imaging, particularly in the detection and management of kidney stones, and suggests avenues for future advancements in automated healthcare systems.