International Journal of Progressive Research in Engineering Management and Science
(Peer-Reviewed, Open Access, Fully Referred International Journal)
www.ijprems.com
editor@ijprems.com or Whatsapp at (+91-9098855509)
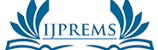
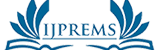
Autocorrection models using Natural Language Processing (KEY IJP************236)
Abstract
This paper presents a comparative study of three prevalent approaches; N-Gram, Hidden Markov Model (HMM), and Rule-Based system in autocorrection systems for Natural Language Processing (NLP). Autocorrection systems are essential tools in digital communication platforms for rectifying typing errors and enhancing user experience. The N-Gram model employs statistical probabilities of word sequences to predict and rectify errors, exhibiting proficiency in local context comprehension but encountering challenges in handling out-of-vocabulary terms and capturing extensive dependencies. In contrast, the Hidden Markov Model excels in modeling sequential data and dependencies between observable and latent states, yet faces obstacles in parameter estimation and scalability. Furthermore, rule-based approaches leverage predefined linguistic rules and patterns for error detection and correction, offering transparency and customization flexibility, but struggling with intricate linguistic phenomena and language variations. Through empirical evaluations on standard datasets, this research compares the performance of these approaches in accuracy, computational efficiency, and robustness across diverse error types and language domains. Additionally, it discusses hybrid methodologies amalgamating the strengths of multiple techniques to enhance autocorrection performance. The findings contribute insights into the strengths and limitations of each approach, guiding future research and advancements in autocorrection systems for NLP applications.
DOI Requested