International Journal of Progressive Research in Engineering Management and Science
(Peer-Reviewed, Open Access, Fully Referred International Journal)
www.ijprems.com
editor@ijprems.com or Whatsapp at (+91-9098855509)
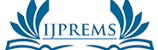
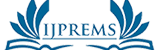
Machine Learning-Based Fitness Tracking Analysis: Classifying Activity Levels and Ensuring Fairness Across Demographics (KEY IJP************657)
Abstract
This study explores the classification of physical activity levelsLow, Moderate, and High using machine learning models based on key health metrics such as step count, calorie intake, sleep duration, and gender. A synthetic dataset of 500 samples was generated to simulate real-world fitness tracking data. Multiple machine learning models, including Random Forest, Logistic Regression, SVM, KNN, and Gradient Boosting, were compared to determine the most effective classification approach.Among the tested models, the Random Forest classifier achieved the highest accuracy (92.3%), demonstrating its superiority in activity level classification. Feature importance analysis identified step count and calorie expenditure as the most influential factors, while sleep duration and gender had a moderate impact. Additionally, bias analysis was conducted to ensure fairness in classification across demographic groups.