International Journal of Progressive Research in Engineering Management and Science
(Peer-Reviewed, Open Access, Fully Referred International Journal)
www.ijprems.com
editor@ijprems.com or Whatsapp at (+91-9098855509)
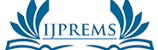
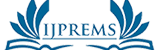
Soil Moisture Retrieval with Vertical and Horizontal polarization Satellite Data Using Deep Learning Models (KEY IJP************136)
Abstract
Soil moisture (SM) is a critical factor for comprehending the interactions and feedback mechanisms between the atmosphere and the Earth's surface, particularly in relation to energy and water cycles. The challenge of accurately determining the spatiotemporal distribution of land surface SM has persisted within the remote sensing field. The model proposed here incorporates various algorithms, including artificial neural networks (ANN), deep neural networks, and three support vector regression (SVR) modelsnamely, radial basis function (SVR_rbf), linear (SVR_linear), and polynomial (SVR_quad) kernelsas well as two tree-based techniques: random forest and eXtreme Gradient Boosting (XGBoost). A comparison of predicted and observed soil moisture values indicated that the most accurate retrievals were achieved using Sentinel-1 data at VV polarization, yielding correlation coefficients (R) between 0.68 and 0.76, along with root-mean-square errors (RMSE) of 0.05 mm and 0.06 mm. Ultimately, SVR_rbf was selected for generating high-resolution soil moisture maps from Sentinel-1 data over irrigated wheat fields, owing to its favorable balance of retrieval accuracy, processing efficiency, and ease of use.Keywords: Machine learning (ML), Deep Learning (DL), Vertical and Horizontal polarization.
DOI Requested